Read Time:
8 min.
Font Size:
Font Weight:
Think Differently to Avoid Silos
The Analytics Continuum
In practical reality, “analytics” comprises many types of analysis, including reporting, visualization, planning, real-time processes, artificial intelligence and machine learning (AI/ML), and natural language processing. The analytics continuum must not only support these analyses, but it must also include the appropriate data management, metadata management and governance capabilities to support access to this information. All these are valuable, each in its own way, and beneficial to different parts of an organization and its operations.
However, one of the most common overall benefits that has been highlighted by participants in our research is that analytics improves communication and knowledge sharing within an organization. That said, today’s organizations must be able to do much more than just share knowledge; they must act on the information they gather and analyze.
Sometimes acting on gathered information is a matter of employing real-time analyses to respond in the moment, before an opportunity is lost. Other possible actions might require sophisticated AI/ML analyses to identify subtle correlations that will allow an organization to predict behaviors or outcomes. Still other actions might involve thorough evaluation of alternative scenarios in order to project the risk and reward trade-offs involved in different outcome scenarios. In all of these, the speed and efficiency of analysis is key.
To this end, advanced technology is now enabling real-time processing. In fact, both B2B and B2C customers have come to expect real-time responsiveness in their customer experiences. Not coincidentally, nearly one-half (46%) of organizations report to us that it is essential to process event data in seconds/sub-seconds. It is worth noting, however, that while visualization may be useful in some situations, it is not a very effective analytical technique for real-time analyses because real-time analyses generally require automation in order to take the required action and generate a timely response.
Technology also enables sophisticated analyses employing AI/ML. More powerful computing infrastructures allow for analysis of greater amounts of data with more complex algorithms, and they are doing it faster than ever before. The automation required in such real-time scenarios requires AI/ML models to recommend the appropriate response based on collected event data. Our research shows that nearly four in ten (38%) organizations are automating their responses to that event data.
Even when the response is not automated, AI/ML-assisted processes can help identify correlations that might not be discovered otherwise, for instance, in finding a process that results in better customer segmentation for sales and marketing activities. AI/ML also drives the use of natural language processing, which can make analytics accessible to a wider audience within organizations. Beyond that, AI/ML can drive the personalization that extends the analytics continuum not just by type of analysis or type of data, but also by the role and habits of the individual.
Data-Driven Organizations Must be Analytics-Driven
There is a lot of talk about “data-driven” organizations. That’s a bit of a misnomer, because what this really means is organizations being analytics-driven. After all, if the collected data is not being used, what’s the point of being data-driven? And an analytics-driven approach requires a continuum.
To support this continuum of analytics, an organization requires a continuum of data capabilities.
To support this continuum of analytics, an organization requires a continuum of data capabilities, and this must be more than a disconnected series of data or analytics siloes. Historical data is necessary to identify trends, detect patterns, and determine seasonality. Planning, then, is typically done at an aggregated level, not at the level of individual transactions. Conversely, AI/ML requires that all detailed data identify correlations that occur specifically at the individual event or transaction level. Finally, real-time analyses require streaming data processing capabilities. A variety of approaches are popular in data retention, but the roiling architectural debate over data warehouses versus data lakes versus lakehouses misses the point of what business units require. All three systems are necessary.
Line of business needs consolidated, cleansed, and organized data that are generally represented in data warehouses. At another level, it also needs non-structured data (images, text, video, logs, device data) that are typically collected in a data lake. Data scientists need the detailed, raw data, which is typically collected in a data lake, not stored in a data warehouse.
But these two worlds are not entirely independent; nearly three-quarters (72%) of the participants in our research report their organization’s data lakes and data warehouses are connected. What we see is that we are in the midst of a paradigm shift from data at rest to data in motion: a continuum.
It was never such that data occurred in isolation or in batches; we only recorded that way because that was what was possible. Those restrictions to batch processing were largely related to technology limitations that no longer exist. And data never really stood still, though the available technology meant we had to collect and analyze as if it were static. It is not and never was.
None of this means that historical data goes away. On the contrary, we still need that data in order to understand the patterns of the past and to be able to extrapolate to current and future scenarios. The world has not caught up entirely—yet—but it is changing rapidly with more options for processing real-time streams of data and events.
Critical Data Capabilities to Enable Lines of Business While Supporting IT
Lines of business need a data infrastructure that provides flexible access to data they can trust. The underlying data infrastructure and BI capabilities must be agile, but more is needed. A continuum of analytics, by its very nature, implies a variety of information brought together from a variety of sources. To operate effectively, organizations need a roadmap to navigate through all that information. The answer is a directory, or catalog, that makes it easy to search for and find the appropriate information.
A catalog is critical to understanding not only what is available, but also to provide the additional context about the data itself, such as its source, its usage, and who is responsible for it. Cataloging easily cuts across the analytics continuum, including the various types of data, various sources and various analytics. The additional information and context provided by the catalog helps individuals develop trust in the data.
One of the biggest challenges of the analytics continuum, however, is supporting ad hoc analyses. Various functions often need to combine different sources of information or bring external or additional sources of information into the analyses. This is where the data marketplace comes into play. The analytics continuum should include mechanisms—a data marketplace—to facilitate and govern the sharing of both internal and external data. Individuals should also be able to share the additional data sources through the data marketplace. Data catalogs and marketplaces also support compliance and governance. They serve that dual purpose, first by making it easy for individuals to access needed information, and then by providing a mechanism for governance and compliance.
Governance and compliance have become increasingly critical issues, especially as our awareness of privacy concerns, regulatory compliance and risk management grows. A strong data foundation is required to meet the requirements for both the lines of business and IT. That foundation, including a data catalog and a data marketplace, can bring together line of business and IT, with compliance and governance in mind, thereby providing value to both parts of the organization.
Analytics and Decision Making Require Collaboration
The analytics continuum must support the communications and collaboration that are integral to analytics processes.
The analytics continuum must support the communications and collaboration that are integral to analytics processes. Analytics and decision-making are rarely conducted by individuals or in isolation. Rather, individuals should be guided through the decision-making process. That means that organizations need to document and share their overall strategy, often by integrating visualizations of key performance indicators via departmental scorecards and strategy maps. Sharing such visualizations and analyses enables clear and consistent communication to those involved in and affected by various decision-making processes. By helping relate specific operations to the overall strategy, these tools equip individuals in the collaborative process.
Supporting collaboration goes beyond sharing visualizations, however. Social media-style collaboration, too, can help document and share the input involved in the decision-making process. In fact, our research shows that nearly nine in ten (89%) organizations already use or intend to use collaboration technology with data and analytics.
Collaborative tools should also support assigning and tracking the actions resulting from the analytics process. Then individuals need to be able to seamlessly incorporate analyses and data into their online discussions. These collaborative streams need to be universally accessible across all types of devices and must include notifications that will alert individuals when their input is required.
Collaborative material from analytics processes can become the foundation for further improvement. For instance, tracking tasks ensures their timely completion and can provide metrics about the resources necessary to implement decisions. Capturing the dialog, decisions, and follow-up provides the basis for additional governance and compliance reporting.
Mining the collaborative dialog can also identify processes requiring further improvement, uncovering inefficiencies, as well as tasks that are not involving the appropriate individuals. Analysis of the collaborative dialogue can even identify to the entire organization valuable resources that might otherwise be underused, such as subject matter experts.
Orchestrating and Coordinating the Analytics Continuum for Agility
Analytics requires the orchestration and coordination of a multistep process. The analytics continuum begins with data operations (DataOps), collecting, preparing, and publishing data for analysis. Analytic operations pick up where DataOps leaves off, executing those analyses and communicating or distributing the results. Machine Learning operations (ML Ops) then orchestrates the process of training, evaluating and deploying AI/ML models. All of these processes need to be coordinated with the development operations (DevOps) associated with maintaining and improving the core business applications. These core applications produce the data that feeds the analytic continuum while also consuming the analyses the continuum produces.
Change is constant in all of this, leading to the requirement for agility in all processes. Gone are the days that organizations could wait months for changes to systems and analyses. Orchestration activities must be designed to recognize and respond to change, and AI/ML will help.
By 2025, 9 in 10 analytics processes will be enhanced by artificial intelligence and machine learning to streamline operations and increase the value that can be derived from data. To be clear, these enhancements are not separate data science exercises. AI/ML will be baked into an organization’s standard data and analytics tools. For example, automatic generation of insights from the data can help direct individuals to the most critical issues. AI/ML can also be used to automatically detect and correct for slight changes in data sources, such as new fields in an application. Larger changes should be data-driven as well, to allow for easy and quick modification. Without such planning and designing for change, organizations will not be as responsive as the market demands.
In the end, the value of analytics is realized not from the analysis, but from the actions of the organization. To maximize value, the analytics continuum needs to connect back to business applications. The continuum needs to be connected to and orchestrated with operational applications in order to capture and implement the results of the analyses.
In some cases, the orchestration can be automated by embedding the analyses, especially AI/ML-based models, into applications. In other cases, the decisions of individuals reviewing and collaborating around the analyses needs to be orchestrated with the applications, making it easier to implement their decisions. In all cases, the value of the analytics continuum is only realized when its components are tied together with the operations of the organization.
Think Differently to Avoid Silos
The Analytics Continuum
In practical reality, “analytics” comprises many types of analysis, including reporting, visualization, planning, real-time processes, artificial intelligence and machine learning (AI/ML), and natural language processing. The analytics continuum must not only support these analyses, but it must also include the appropriate data management, metadata management and governance capabilities to support access to this information. All these are valuable, each in its own way, and beneficial to different parts of an organization and its operations.
However, one of the most common overall benefits that has been highlighted by participants in our research is that analytics improves communication and knowledge sharing within an organization. That said, today’s organizations must be able to do much more than just share knowledge; they must act on the information they gather and analyze.
Sometimes acting on gathered information is a matter of employing real-time analyses to respond in the moment, before an opportunity is lost. Other possible actions might require sophisticated AI/ML analyses to identify subtle correlations that will allow an organization to predict behaviors or outcomes. Still other actions might involve thorough evaluation of alternative scenarios in order to project the risk and reward trade-offs involved in different outcome scenarios. In all of these, the speed and efficiency of analysis is key.
To this end, advanced technology is now enabling real-time processing. In fact, both B2B and B2C customers have come to expect real-time responsiveness in their customer experiences. Not coincidentally, nearly one-half (46%) of organizations report to us that it is essential to process event data in seconds/sub-seconds. It is worth noting, however, that while visualization may be useful in some situations, it is not a very effective analytical technique for real-time analyses because real-time analyses generally require automation in order to take the required action and generate a timely response.
Technology also enables sophisticated analyses employing AI/ML. More powerful computing infrastructures allow for analysis of greater amounts of data with more complex algorithms, and they are doing it faster than ever before. The automation required in such real-time scenarios requires AI/ML models to recommend the appropriate response based on collected event data. Our research shows that nearly four in ten (38%) organizations are automating their responses to that event data.
Even when the response is not automated, AI/ML-assisted processes can help identify correlations that might not be discovered otherwise, for instance, in finding a process that results in better customer segmentation for sales and marketing activities. AI/ML also drives the use of natural language processing, which can make analytics accessible to a wider audience within organizations. Beyond that, AI/ML can drive the personalization that extends the analytics continuum not just by type of analysis or type of data, but also by the role and habits of the individual.
Data-Driven Organizations Must be Analytics-Driven
There is a lot of talk about “data-driven” organizations. That’s a bit of a misnomer, because what this really means is organizations being analytics-driven. After all, if the collected data is not being used, what’s the point of being data-driven? And an analytics-driven approach requires a continuum.
To support this continuum of analytics, an organization requires a continuum of data capabilities.
To support this continuum of analytics, an organization requires a continuum of data capabilities, and this must be more than a disconnected series of data or analytics siloes. Historical data is necessary to identify trends, detect patterns, and determine seasonality. Planning, then, is typically done at an aggregated level, not at the level of individual transactions. Conversely, AI/ML requires that all detailed data identify correlations that occur specifically at the individual event or transaction level. Finally, real-time analyses require streaming data processing capabilities. A variety of approaches are popular in data retention, but the roiling architectural debate over data warehouses versus data lakes versus lakehouses misses the point of what business units require. All three systems are necessary.
Line of business needs consolidated, cleansed, and organized data that are generally represented in data warehouses. At another level, it also needs non-structured data (images, text, video, logs, device data) that are typically collected in a data lake. Data scientists need the detailed, raw data, which is typically collected in a data lake, not stored in a data warehouse.
But these two worlds are not entirely independent; nearly three-quarters (72%) of the participants in our research report their organization’s data lakes and data warehouses are connected. What we see is that we are in the midst of a paradigm shift from data at rest to data in motion: a continuum.
It was never such that data occurred in isolation or in batches; we only recorded that way because that was what was possible. Those restrictions to batch processing were largely related to technology limitations that no longer exist. And data never really stood still, though the available technology meant we had to collect and analyze as if it were static. It is not and never was.
None of this means that historical data goes away. On the contrary, we still need that data in order to understand the patterns of the past and to be able to extrapolate to current and future scenarios. The world has not caught up entirely—yet—but it is changing rapidly with more options for processing real-time streams of data and events.
Critical Data Capabilities to Enable Lines of Business While Supporting IT
Lines of business need a data infrastructure that provides flexible access to data they can trust. The underlying data infrastructure and BI capabilities must be agile, but more is needed. A continuum of analytics, by its very nature, implies a variety of information brought together from a variety of sources. To operate effectively, organizations need a roadmap to navigate through all that information. The answer is a directory, or catalog, that makes it easy to search for and find the appropriate information.
A catalog is critical to understanding not only what is available, but also to provide the additional context about the data itself, such as its source, its usage, and who is responsible for it. Cataloging easily cuts across the analytics continuum, including the various types of data, various sources and various analytics. The additional information and context provided by the catalog helps individuals develop trust in the data.
One of the biggest challenges of the analytics continuum, however, is supporting ad hoc analyses. Various functions often need to combine different sources of information or bring external or additional sources of information into the analyses. This is where the data marketplace comes into play. The analytics continuum should include mechanisms—a data marketplace—to facilitate and govern the sharing of both internal and external data. Individuals should also be able to share the additional data sources through the data marketplace. Data catalogs and marketplaces also support compliance and governance. They serve that dual purpose, first by making it easy for individuals to access needed information, and then by providing a mechanism for governance and compliance.
Governance and compliance have become increasingly critical issues, especially as our awareness of privacy concerns, regulatory compliance and risk management grows. A strong data foundation is required to meet the requirements for both the lines of business and IT. That foundation, including a data catalog and a data marketplace, can bring together line of business and IT, with compliance and governance in mind, thereby providing value to both parts of the organization.
Analytics and Decision Making Require Collaboration
The analytics continuum must support the communications and collaboration that are integral to analytics processes.
The analytics continuum must support the communications and collaboration that are integral to analytics processes. Analytics and decision-making are rarely conducted by individuals or in isolation. Rather, individuals should be guided through the decision-making process. That means that organizations need to document and share their overall strategy, often by integrating visualizations of key performance indicators via departmental scorecards and strategy maps. Sharing such visualizations and analyses enables clear and consistent communication to those involved in and affected by various decision-making processes. By helping relate specific operations to the overall strategy, these tools equip individuals in the collaborative process.
Supporting collaboration goes beyond sharing visualizations, however. Social media-style collaboration, too, can help document and share the input involved in the decision-making process. In fact, our research shows that nearly nine in ten (89%) organizations already use or intend to use collaboration technology with data and analytics.
Collaborative tools should also support assigning and tracking the actions resulting from the analytics process. Then individuals need to be able to seamlessly incorporate analyses and data into their online discussions. These collaborative streams need to be universally accessible across all types of devices and must include notifications that will alert individuals when their input is required.
Collaborative material from analytics processes can become the foundation for further improvement. For instance, tracking tasks ensures their timely completion and can provide metrics about the resources necessary to implement decisions. Capturing the dialog, decisions, and follow-up provides the basis for additional governance and compliance reporting.
Mining the collaborative dialog can also identify processes requiring further improvement, uncovering inefficiencies, as well as tasks that are not involving the appropriate individuals. Analysis of the collaborative dialogue can even identify to the entire organization valuable resources that might otherwise be underused, such as subject matter experts.
Orchestrating and Coordinating the Analytics Continuum for Agility
Analytics requires the orchestration and coordination of a multistep process. The analytics continuum begins with data operations (DataOps), collecting, preparing, and publishing data for analysis. Analytic operations pick up where DataOps leaves off, executing those analyses and communicating or distributing the results. Machine Learning operations (ML Ops) then orchestrates the process of training, evaluating and deploying AI/ML models. All of these processes need to be coordinated with the development operations (DevOps) associated with maintaining and improving the core business applications. These core applications produce the data that feeds the analytic continuum while also consuming the analyses the continuum produces.
Change is constant in all of this, leading to the requirement for agility in all processes. Gone are the days that organizations could wait months for changes to systems and analyses. Orchestration activities must be designed to recognize and respond to change, and AI/ML will help.
By 2025, 9 in 10 analytics processes will be enhanced by artificial intelligence and machine learning to streamline operations and increase the value that can be derived from data. To be clear, these enhancements are not separate data science exercises. AI/ML will be baked into an organization’s standard data and analytics tools. For example, automatic generation of insights from the data can help direct individuals to the most critical issues. AI/ML can also be used to automatically detect and correct for slight changes in data sources, such as new fields in an application. Larger changes should be data-driven as well, to allow for easy and quick modification. Without such planning and designing for change, organizations will not be as responsive as the market demands.
In the end, the value of analytics is realized not from the analysis, but from the actions of the organization. To maximize value, the analytics continuum needs to connect back to business applications. The continuum needs to be connected to and orchestrated with operational applications in order to capture and implement the results of the analyses.
In some cases, the orchestration can be automated by embedding the analyses, especially AI/ML-based models, into applications. In other cases, the decisions of individuals reviewing and collaborating around the analyses needs to be orchestrated with the applications, making it easier to implement their decisions. In all cases, the value of the analytics continuum is only realized when its components are tied together with the operations of the organization.
Fill out the form to continue reading
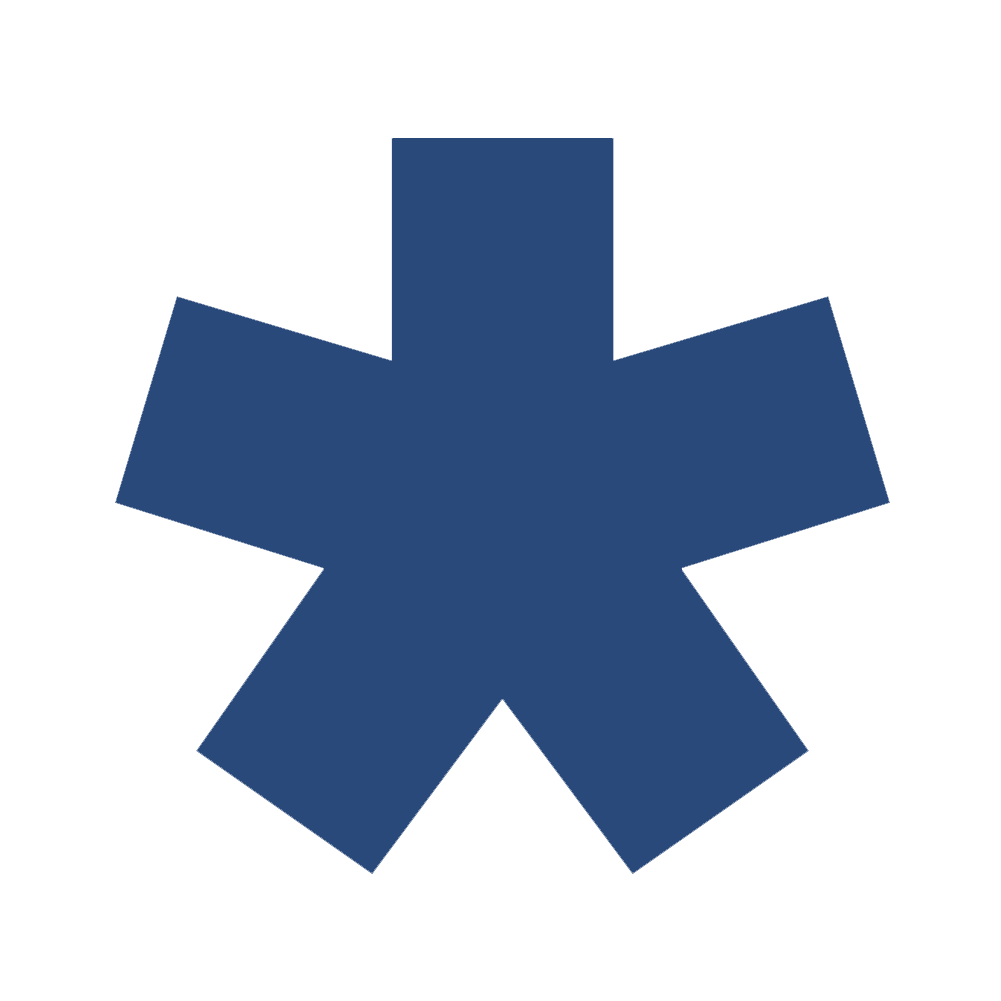
ISG Software Research
ISG Software Research is the most authoritative and respected market research and advisory services firm focused on improving business outcomes through optimal use of people, processes, information and technology. Since our beginning, our goal has been to provide insight and expert guidance on mainstream and disruptive technologies. In short, we want to help you become smarter and find the most relevant technology to accelerate your organization's goals.
About ISG Software Research
ISG Software Research provides expert market insights on vertical industries, business, AI and IT through comprehensive consulting, advisory and research services with world-class industry analysts and client experience. Our ISG Buyers Guides offer comprehensive ratings and insights into technology providers and products. Explore our research at research.isg-one.com.
About ISG Research
ISG Research provides subscription research, advisory consulting and executive event services focused on market trends and disruptive technologies driving change in business computing. ISG Research delivers guidance that helps businesses accelerate growth and create more value. For more information about ISG Research subscriptions, please email contact@isg-one.com.
About ISG
ISG (Information Services Group) (Nasdaq: III) is a leading global technology research and advisory firm. A trusted business partner to more than 900 clients, including more than 75 of the world’s top 100 enterprises, ISG is committed to helping corporations, public sector organizations, and service and technology providers achieve operational excellence and faster growth. The firm specializes in digital transformation services, including AI and automation, cloud and data analytics; sourcing advisory; managed governance and risk services; network carrier services; strategy and operations design; change management; market intelligence and technology research and analysis. Founded in 2006 and based in Stamford, Conn., ISG employs 1,600 digital-ready professionals operating in more than 20 countries—a global team known for its innovative thinking, market influence, deep industry and technology expertise, and world-class research and analytical capabilities based on the industry’s most comprehensive marketplace data.
For more information, visit isg-one.com.