Read Time:
8 min.
Sponsored by:
Font Size:
Font Weight:
AI-Powered Data Unification for Successful Data-Driven Initiatives
Better Trust in Data for Better Use of Data
The current economic climate has highlighted more than ever the differences between enterprises that can turn data into actionable insights and those that are incapable of seeing or responding to the need for change. Data-driven enterprises stand to gain a competitive advantage by increasing the speed and scale at which data is accessed and processed. One obstacle they face is that core data sets from various organizations within the enterprise, such as finance, human resources or supply chain, can become siloed within applications. Extracting data from these applications and transforming and loading it into a data warehouse makes it available for analysis for multiple purposes, but this can be a time-consuming process that delays business insight. Additionally, data duplication and fragmentation issues can lead to disagreements across the business about data definitions and accuracy. To improve business agility, enterprises need access to linked and comprehensive data that is available in real time and that they can trust to be valid, consistent and current.
Having trust in data is critical to the ability of an enterprise to make data-driven business decisions and accelerate digital transformation initiatives. Data leaders need to be able to deliver trusted, reusable, up-to-date data sets for operational and analytics initiatives led by business teams and data scientists. They also must ensure data access is democratized without sacrificing data security and governance. Analytics and data science teams need quick access to trusted data sets with flexible modeling and rich attributes that are continuously curated. They also need to reduce the time and effort spent on data preparation and have well-governed data to improve machine learning (ML) governance and transparency. However, only 28% of participants in our Data Governance Benchmark Research say data is well-trusted in their organization. As a result, it is not surprising that two-thirds of enterprises are not very confident in governing data across the business. Having confidence in the data used to run and analyze the business requires real-time access to trusted data from multiple applications and use cases.
Ensuring the integrity of data used for business decision-making can be difficult given that organizations have an increasing volume and range of data sources to contend with. Data unification, data quality and master data management (MDM) technologies and practices enable enterprises to ensure data is accurate, complete and consistent. Despite the availability of technology to unify data in real-time, many enterprises erroneously believe that implementing a unified, 360-degree view of core data that is curated on a continuous basis and available to match the speed of business operations is too complex and too costly.
Data Unification Requires Understanding Data Flows
Data silos are an inevitable consequence of the critical importance of applications in use by core departments such as office of finance, sales, customer service and supply chain and the deployment of dedicated data platforms for each use case. The rapid adoption of software-as-a-service (SaaS) applications and cloud computing has further fragmented the storage and processing of data, which is increasingly spread across multiple data centers and cloud providers. By 2026, 8 in 10 enterprises will have data spread across multiple cloud providers and on-premises data centers, requiring investment in data management products that span multiple locations. Traditional approaches to data integration enable enterprises to combine multiple data sources for operational or analytics initiatives, but each integration initiative creates another version of the data with its own data formats and definitions. Alternatively, data unification strategies enable enterprises to overcome the challenges presented by distributed data by facilitating data integrity, promoting trust in data and supporting interoperability across multiple business units and use-cases.
A broad, strategic data intelligence initiative relies on having a solid understanding of how, when and why data is produced and consumed across the organization, by whom, and for what purpose. Data intelligence combines capabilities related to data integration, data catalog, data quality, data lineage, metadata management and MDM to facilitate this understanding. This is critical to rectifying previously disjointed approaches to data production and data consumption. As a result, through 2027, three-quarters of enterprises will be engaged in data intelligence initiatives to increase trust in their data by utilizing metadata to understand how, when, where and why data is used in their organization, and by whom.
Taking a proactive approach to data unification can provide well-governed data and accelerate its delivery by removing barriers that prevent or delay users from gaining access to data. This enables data to be treated as a product that is generated and consumed by multiple internal business units, or by external partners and customers. This is particularly important for enterprises adopting the data mesh approach to data ownership, access and governance. By 2027, more than 6 in 10 enterprises will adopt technologies to facilitate the delivery of data as a product as they adapt their cultural and organizational approaches to data ownership in the context of data mesh.
Democratized Access to Trusted Data
Data mesh and data fabric are two of the current approaches to data management and operations that provide timely access to trusted data and reduce costs and complexity. Despite often being used interchangeably, data fabric and data mesh relate to independent but intersecting concepts. Data fabric is a technology-driven approach to managing and governing data across distributed environments, while data mesh is an organizational and cultural approach to data ownership, access and governance. Data fabric is differentiated by its focus on how data is produced—specifically, the tools and technologies primarily aimed at data management and governance that practitioners use to deliver agile data integration. In comparison, data mesh is more concerned with how data is consumed by business users. Domain-oriented data ownership is integral to data mesh, with the business departments or units that generate the data having responsibility for managing ownership of, and access to, the data.
Data fabric and data mesh both rely on and facilitate self-service data access and federated governance.
The two approaches are complementary. Data fabric and data mesh both rely on and facilitate self-service data access and federated governance. Data fabric can provide the underlying data integration and management capabilities that enable data management professionals to deliver self-service data access, federated data governance and data as a product, while data mesh utilizes federated data governance and domain-oriented data ownership to encourage business users to consume data as a product via self-service data access.
Many organizations see data catalogs as the solution to poor data governance and the need for data democratization because they facilitate self-service access to data. But self-service access to data is only truly valuable if users can trust the data they have access to. Our Data Governance Benchmark Research indicates that data quality issues and multiple versions of the truth are the barriers to data governance that have the greatest impact on business operations. Pulling data from multiple data sources into a single data catalog does not in itself provide a single version of the truth that can be relied upon for business decision-making across an enterprise.
Making data available as a product on a self-service basis increases the importance of agreed-upon data definitions and entity resolution. One-half of participants in our Data Governance Benchmark Research cite agreement on the definitions of data as a primary concern in managing data effectively. Reaching agreement on an enterprise’s core data entities is the focus of MDM. “Master data” is the term used for an organization’s foundational reference data, and it provides an agreed-to list of entities that can be shared throughout the enterprise, including categories such as parties (customers or workers), places (addresses or regions) and things (products, assets, financial instruments). Master data management is the practice of managing that master data and encompasses processes such as data validation, matching and merging duplicate records and enriching data with related information. Another important component is data modeling, which documents the relationships between data elements. This results in the generation of an enterprise glossary for use across the organization, as well as by partners and suppliers, and utilized by data catalogs, providing shared trust in the validity of the data.
Business Benefits of Data Unification
A comprehensive, real time and trusted 360-degree view of data enabled by data unification can provide support for multiple enterprise business initiatives, including machine learning, by providing rich attributes and a holistic view of business interactions and relationships. This enables enterprises to create interoperable data products for different business units and use cases without duplicating time, effort or data.
MDM is a core capability within any data unification initiative, facilitating a 360-degree view of customers, partners and suppliers by providing common definitions across multiple data sources. More than 8 in 10 participants (82%) in Ventana Research’s Data Governance Benchmark Research are using MDM technologies for data governance, increasing their trust in data. Almost three-quarters of enterprises that use MDM for data governance (73%) are confident in their organization’s ability to govern and manage data across the business, compared to only 27% of those that do not use MDM for data governance. Data-savvy organizations seek out data unification products with multi-domain MDM capabilities, aligning with data fabric and data mesh architectural approaches and providing the functionality to address customer and product data alongside data about employees, assets, suppliers, locations and any other pertinent business data. Enterprises that have employed a robust data unification initiative benefit from a unified, trusted, up-to-date view of all core data domains, enabling interoperability and accelerating the delivery of data products that more rapidly provide business stakeholders with high-quality data used to drive value through efficiency and improved customer engagement. This puts them in a better position to not only retain customers and sell more products, but to accelerate data products and respond to evolving business requirements. The efficiency and effectiveness of that response is further increased when automation is introduced to the data unification process.
Artificial Intelligence Enhances Data Unification
The use of artificial intelligence and machine learning (AI/ML) in data unification initiatives can enhance multiple aspects of data unification, including fundamental entity resolution and matching capabilities. It can also aid with mastering multiple domains and gaining a comprehensive 360-degree view of transactions, interactions and relationships. Specifically, AI/ML can be used to automatically identify missing or inaccurate relationships in data that might otherwise have been overlooked in manual processes. Additionally, multiple matching algorithms combined with ML scoring capabilities can help improve the accuracy of data-matching. ML techniques can also be used to identify and provide access to information most likely to be relevant to a specific user and their role, providing guided authoring and assistance, automating data profiling processes, and recommending related information from multiple domains in the data governance process.
Key Takeaways
- Organizations with higher levels of confidence in data can move more quickly to make data-driven decisions, responding faster to knowledge worker and customer demands for more innovative, data-rich applications and personalized experiences, thus gaining competitive advantage.
- Data unification products with AI capabilities can lower the barriers to successful data governance and sharing and accelerate time to value.
- Organizations that aspire to operational excellence and decision-making driven by trusted data should evaluate the new breed of data unification products with a view to increasing trust in data and data management processes.
AI-Powered Data Unification for Successful Data-Driven Initiatives
Better Trust in Data for Better Use of Data
The current economic climate has highlighted more than ever the differences between enterprises that can turn data into actionable insights and those that are incapable of seeing or responding to the need for change. Data-driven enterprises stand to gain a competitive advantage by increasing the speed and scale at which data is accessed and processed. One obstacle they face is that core data sets from various organizations within the enterprise, such as finance, human resources or supply chain, can become siloed within applications. Extracting data from these applications and transforming and loading it into a data warehouse makes it available for analysis for multiple purposes, but this can be a time-consuming process that delays business insight. Additionally, data duplication and fragmentation issues can lead to disagreements across the business about data definitions and accuracy. To improve business agility, enterprises need access to linked and comprehensive data that is available in real time and that they can trust to be valid, consistent and current.
Having trust in data is critical to the ability of an enterprise to make data-driven business decisions and accelerate digital transformation initiatives. Data leaders need to be able to deliver trusted, reusable, up-to-date data sets for operational and analytics initiatives led by business teams and data scientists. They also must ensure data access is democratized without sacrificing data security and governance. Analytics and data science teams need quick access to trusted data sets with flexible modeling and rich attributes that are continuously curated. They also need to reduce the time and effort spent on data preparation and have well-governed data to improve machine learning (ML) governance and transparency. However, only 28% of participants in our Data Governance Benchmark Research say data is well-trusted in their organization. As a result, it is not surprising that two-thirds of enterprises are not very confident in governing data across the business. Having confidence in the data used to run and analyze the business requires real-time access to trusted data from multiple applications and use cases.
Ensuring the integrity of data used for business decision-making can be difficult given that organizations have an increasing volume and range of data sources to contend with. Data unification, data quality and master data management (MDM) technologies and practices enable enterprises to ensure data is accurate, complete and consistent. Despite the availability of technology to unify data in real-time, many enterprises erroneously believe that implementing a unified, 360-degree view of core data that is curated on a continuous basis and available to match the speed of business operations is too complex and too costly.
Data Unification Requires Understanding Data Flows
Data silos are an inevitable consequence of the critical importance of applications in use by core departments such as office of finance, sales, customer service and supply chain and the deployment of dedicated data platforms for each use case. The rapid adoption of software-as-a-service (SaaS) applications and cloud computing has further fragmented the storage and processing of data, which is increasingly spread across multiple data centers and cloud providers. By 2026, 8 in 10 enterprises will have data spread across multiple cloud providers and on-premises data centers, requiring investment in data management products that span multiple locations. Traditional approaches to data integration enable enterprises to combine multiple data sources for operational or analytics initiatives, but each integration initiative creates another version of the data with its own data formats and definitions. Alternatively, data unification strategies enable enterprises to overcome the challenges presented by distributed data by facilitating data integrity, promoting trust in data and supporting interoperability across multiple business units and use-cases.
A broad, strategic data intelligence initiative relies on having a solid understanding of how, when and why data is produced and consumed across the organization, by whom, and for what purpose. Data intelligence combines capabilities related to data integration, data catalog, data quality, data lineage, metadata management and MDM to facilitate this understanding. This is critical to rectifying previously disjointed approaches to data production and data consumption. As a result, through 2027, three-quarters of enterprises will be engaged in data intelligence initiatives to increase trust in their data by utilizing metadata to understand how, when, where and why data is used in their organization, and by whom.
Taking a proactive approach to data unification can provide well-governed data and accelerate its delivery by removing barriers that prevent or delay users from gaining access to data. This enables data to be treated as a product that is generated and consumed by multiple internal business units, or by external partners and customers. This is particularly important for enterprises adopting the data mesh approach to data ownership, access and governance. By 2027, more than 6 in 10 enterprises will adopt technologies to facilitate the delivery of data as a product as they adapt their cultural and organizational approaches to data ownership in the context of data mesh.
Democratized Access to Trusted Data
Data mesh and data fabric are two of the current approaches to data management and operations that provide timely access to trusted data and reduce costs and complexity. Despite often being used interchangeably, data fabric and data mesh relate to independent but intersecting concepts. Data fabric is a technology-driven approach to managing and governing data across distributed environments, while data mesh is an organizational and cultural approach to data ownership, access and governance. Data fabric is differentiated by its focus on how data is produced—specifically, the tools and technologies primarily aimed at data management and governance that practitioners use to deliver agile data integration. In comparison, data mesh is more concerned with how data is consumed by business users. Domain-oriented data ownership is integral to data mesh, with the business departments or units that generate the data having responsibility for managing ownership of, and access to, the data.
Data fabric and data mesh both rely on and facilitate self-service data access and federated governance.
The two approaches are complementary. Data fabric and data mesh both rely on and facilitate self-service data access and federated governance. Data fabric can provide the underlying data integration and management capabilities that enable data management professionals to deliver self-service data access, federated data governance and data as a product, while data mesh utilizes federated data governance and domain-oriented data ownership to encourage business users to consume data as a product via self-service data access.
Many organizations see data catalogs as the solution to poor data governance and the need for data democratization because they facilitate self-service access to data. But self-service access to data is only truly valuable if users can trust the data they have access to. Our Data Governance Benchmark Research indicates that data quality issues and multiple versions of the truth are the barriers to data governance that have the greatest impact on business operations. Pulling data from multiple data sources into a single data catalog does not in itself provide a single version of the truth that can be relied upon for business decision-making across an enterprise.
Making data available as a product on a self-service basis increases the importance of agreed-upon data definitions and entity resolution. One-half of participants in our Data Governance Benchmark Research cite agreement on the definitions of data as a primary concern in managing data effectively. Reaching agreement on an enterprise’s core data entities is the focus of MDM. “Master data” is the term used for an organization’s foundational reference data, and it provides an agreed-to list of entities that can be shared throughout the enterprise, including categories such as parties (customers or workers), places (addresses or regions) and things (products, assets, financial instruments). Master data management is the practice of managing that master data and encompasses processes such as data validation, matching and merging duplicate records and enriching data with related information. Another important component is data modeling, which documents the relationships between data elements. This results in the generation of an enterprise glossary for use across the organization, as well as by partners and suppliers, and utilized by data catalogs, providing shared trust in the validity of the data.
Business Benefits of Data Unification
A comprehensive, real time and trusted 360-degree view of data enabled by data unification can provide support for multiple enterprise business initiatives, including machine learning, by providing rich attributes and a holistic view of business interactions and relationships. This enables enterprises to create interoperable data products for different business units and use cases without duplicating time, effort or data.
MDM is a core capability within any data unification initiative, facilitating a 360-degree view of customers, partners and suppliers by providing common definitions across multiple data sources. More than 8 in 10 participants (82%) in Ventana Research’s Data Governance Benchmark Research are using MDM technologies for data governance, increasing their trust in data. Almost three-quarters of enterprises that use MDM for data governance (73%) are confident in their organization’s ability to govern and manage data across the business, compared to only 27% of those that do not use MDM for data governance. Data-savvy organizations seek out data unification products with multi-domain MDM capabilities, aligning with data fabric and data mesh architectural approaches and providing the functionality to address customer and product data alongside data about employees, assets, suppliers, locations and any other pertinent business data. Enterprises that have employed a robust data unification initiative benefit from a unified, trusted, up-to-date view of all core data domains, enabling interoperability and accelerating the delivery of data products that more rapidly provide business stakeholders with high-quality data used to drive value through efficiency and improved customer engagement. This puts them in a better position to not only retain customers and sell more products, but to accelerate data products and respond to evolving business requirements. The efficiency and effectiveness of that response is further increased when automation is introduced to the data unification process.
Artificial Intelligence Enhances Data Unification
The use of artificial intelligence and machine learning (AI/ML) in data unification initiatives can enhance multiple aspects of data unification, including fundamental entity resolution and matching capabilities. It can also aid with mastering multiple domains and gaining a comprehensive 360-degree view of transactions, interactions and relationships. Specifically, AI/ML can be used to automatically identify missing or inaccurate relationships in data that might otherwise have been overlooked in manual processes. Additionally, multiple matching algorithms combined with ML scoring capabilities can help improve the accuracy of data-matching. ML techniques can also be used to identify and provide access to information most likely to be relevant to a specific user and their role, providing guided authoring and assistance, automating data profiling processes, and recommending related information from multiple domains in the data governance process.
Key Takeaways
- Organizations with higher levels of confidence in data can move more quickly to make data-driven decisions, responding faster to knowledge worker and customer demands for more innovative, data-rich applications and personalized experiences, thus gaining competitive advantage.
- Data unification products with AI capabilities can lower the barriers to successful data governance and sharing and accelerate time to value.
- Organizations that aspire to operational excellence and decision-making driven by trusted data should evaluate the new breed of data unification products with a view to increasing trust in data and data management processes.
Fill out the form to continue reading
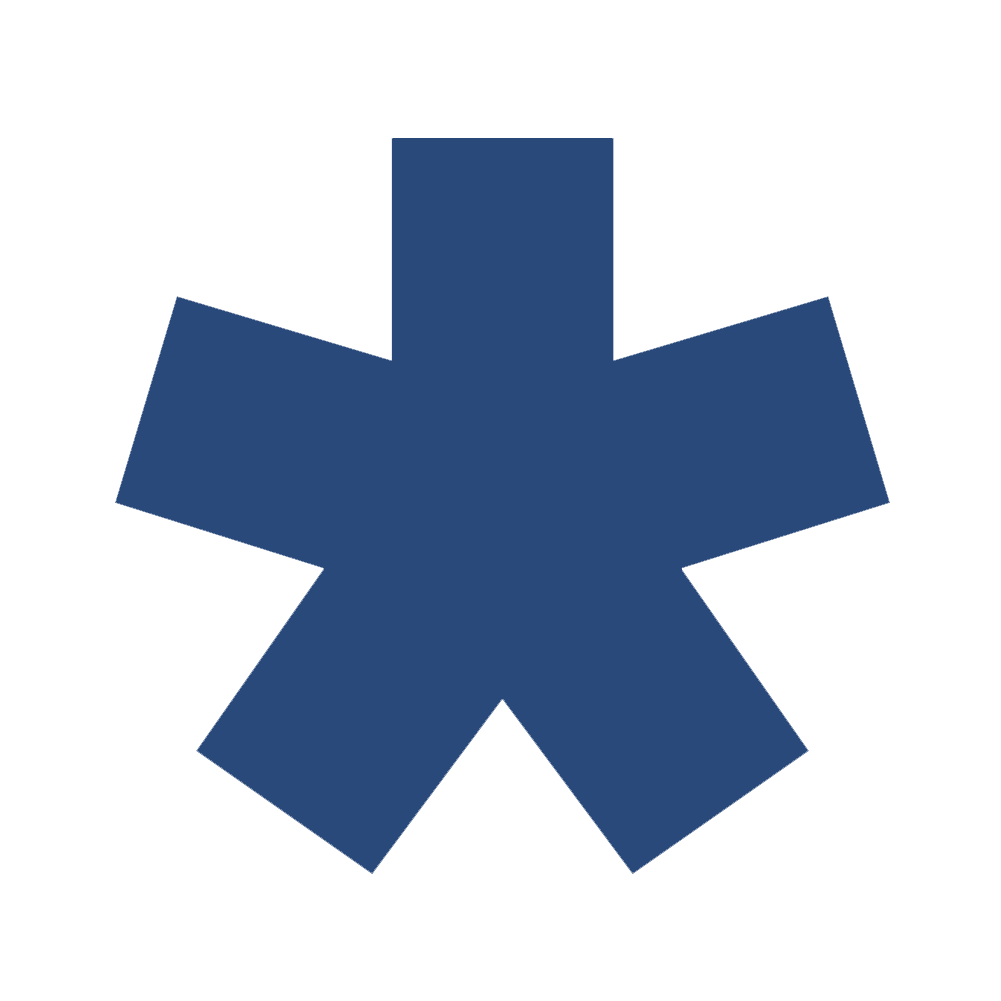
ISG Software Research
ISG Software Research is the most authoritative and respected market research and advisory services firm focused on improving business outcomes through optimal use of people, processes, information and technology. Since our beginning, our goal has been to provide insight and expert guidance on mainstream and disruptive technologies. In short, we want to help you become smarter and find the most relevant technology to accelerate your organization's goals.
About ISG Software Research
ISG Software Research provides expert market insights on vertical industries, business, AI and IT through comprehensive consulting, advisory and research services with world-class industry analysts and client experience. Our ISG Buyers Guides offer comprehensive ratings and insights into technology providers and products. Explore our research at research.isg-one.com.
About ISG Research
ISG Research provides subscription research, advisory consulting and executive event services focused on market trends and disruptive technologies driving change in business computing. ISG Research delivers guidance that helps businesses accelerate growth and create more value. For more information about ISG Research subscriptions, please email contact@isg-one.com.
About ISG
ISG (Information Services Group) (Nasdaq: III) is a leading global technology research and advisory firm. A trusted business partner to more than 900 clients, including more than 75 of the world’s top 100 enterprises, ISG is committed to helping corporations, public sector organizations, and service and technology providers achieve operational excellence and faster growth. The firm specializes in digital transformation services, including AI and automation, cloud and data analytics; sourcing advisory; managed governance and risk services; network carrier services; strategy and operations design; change management; market intelligence and technology research and analysis. Founded in 2006 and based in Stamford, Conn., ISG employs 1,600 digital-ready professionals operating in more than 20 countries—a global team known for its innovative thinking, market influence, deep industry and technology expertise, and world-class research and analytical capabilities based on the industry’s most comprehensive marketplace data.
For more information, visit isg-one.com.