Read Time:
8 min.
Sponsored by:
Font Size:
Font Weight:
Accelerate Enterprise Data Science in the Hybrid Cloud with MLOps
Overcome the Challenges of Operationalizing AI/ML
Data is an extremely valuable asset for every organization, but it is meaningless until it is used to make actionable decisions. Given the volume of data generated and collected today, using artificial intelligence with machine learning (AI/ML) is the most efficient way for organizations to sift through data and extract value. All industries and line-of-business functions find value by integrating AI/ML into data science efforts. Among participants in our Analytics and Data Benchmark Research, 97% of financial services organizations reported that AI/ML is important or very important, particularly for detecting and preventing fraud. Three-quarters (76%) of technology organizations also reported AI/ML is important or very important. AI/ML is often deployed to prevent cybersecurity disruptions. And more than one-half (57%) of healthcare and life sciences organizations rated AI/ML important or very important Healthcare and life science organizations use AI/ML models for numerous use cases including improving drug discoveries and creating personalized treatments to provide the best possible clinical outcomes for individuals.
However, creating, deploying and managing AI/ML models can be challenging for data and analytics executives. Our research shows that less than one in 10 organizations consider their organization’s AI/ML technology completely adequate and more than two-thirds consider it less than adequate. AI/ML presents several challenges. AI/ML requires a significant amount of data and therefore a scalable, high-performance infrastructure. It also requires a blend of data science skills with software development and operations knowledge. The tools and skills needed to code AI/ML models often do not help when dealing with the infrastructure for deploying and managing those models. Given these challenges, data and analytics executives should carefully weigh the following five considerations for AI initiatives.
1. Scaling Data Science with MLOps and GPUs
Machine learning operations (MLOps) platforms orchestrate the collection of artifacts, compute infrastructure, regardless of location, and processes necessary to deploy and maintain AI-based models. MLOps platforms facilitate close interaction between data science, application development and operations to increase productivity and deploy a greater number of models into production quickly. These platforms provide governance over access to models and the features associated with them. They help ensure models are kept up to date, detecting drift and retraining and redeploying as necessary. They also help detect and prevent bias in the models that are being developed and deployed.
Platforms that share infrastructure among multiple teams and projects help organizations enhance return on investment.
MLOps platforms also remove DevOps barriers for data scientists by providing governed access to the specialized, high-performance infrastructures powered by graphics processing units provide the horsepower needed for AI/ML workloads. GPUs, originally developed for accelerating graphics processing, provide highly parallel processing that accelerates model development and scales deployment, increasing performance and reducing cost. Given the volume of data transfers in model training, high-bandwidth memory and fast networking also help speed training iterations and time to insight.
Productivity of data science resources is critical. Platforms that share infrastructure among multiple teams and projects help organizations enhance return on investment. Increasingly, this type of specialized infrastructure is available as “chips in the cloud” as well as on-premises. While MLOps platforms are the foundation for data science at scale and can get more models into production quickly, siloed data and infrastructure often present unique challenges that disrupt the ML life cycle. This not only reduces productivity, but increases cost, wasted effort, and risk through sub-optimal model performance.
2. Managing Distributed Data On-Premises and in the Cloud
It is common for an organization to work with massive amounts of disparate, distributed data. Data is generated from business processes and gathered from systems and applications as well as sensors and devices used by the organization. Additional data is collected directly from interactions with customers, such as call logs, emails and social media posts. These more complex data sets, especially from new sources, include unstructured data, semi-structured data and external data, and must be managed across cloud services and on-premises data centers.
Through our Analytics and Data Benchmark Research, we found that more than one-half of organizations (56%) access 11 or more data sources, up from 39% four years ago. One-third of participants (32%) report using more than 20 data sources. And, as petabyte size databases become more common, more than one-half of organizations (58%) consider themselves to be using big data, up from 41% in our previous study. Understandably, nearly one-third of organizations (31%) report that it is hard to access data sources and it is the most common challenge cited in applying AI/ML.
Managed cloud services offer organizations many benefits, but on-premises data isn’t going away any time soon. Our research found that 86% of organizations either already have or expect to have the majority of data in the cloud, however on-premises deployments continue to be important to many organizations. Our research shows that more than one-half of organizations (52%) deploy their analytics and data technologies using a hybrid of on-premises and cloud platforms. By 2025, more than three-quarters of enterprises will have data spread across multiple cloud providers and on-premises data centers, requiring investment in data management products that span multiple locations.
There are several factors to consider when managing costs associated with data. Cloud providers charge ingress and egress fees, so it is no longer free to access your data. Currently, extracting and analyzing a petabyte of data could cost as much as $50,000. The cloud also requires internet connectivity to operate, which can lead to latency. Real-time analytics, databases, security applications, software connected to sensors and the IoT are subject to data gravity, creating bigger compute workloads to transmit. The split between on-premises and cloud storage can also cause price complexities.
3. Harnessing Data Gravity with a Hybrid Cloud Strategy
Data gravity is the tendency for big data to attract additional data, services and applications. The larger the data, the greater its gravity. This also relates to the data’s relative permanence, or “heaviness,” and the type of resources required to move it. While cloud architectures provide many benefits, as data grows, data gravity becomes more of an obstacle, providing new challenges for IT leaders.
Privacy regulations have renewed focus on data residency and data sovereignty, particularly with fractured data spread across multiple locations. Data residency refers to the physical location of the data, while sovereignty is the fact that data is regulated by the laws of the country in which it is collected and processed. Managing data sovereignty has become more difficult as analytic systems and data sources have become more distributed.
Through 2026, nearly all multinational organizations will invest in local data processing infrastructure and services to mitigate against the risks associated with data transfer.
Often these data sets are subject to privacy regulations meaning they must stay locked in a single geography. Cloud providers have taken steps to meet government and industry requirements, including for Health Insurance Portability and Accountability Act-compliant information, but not all providers support requirements across all geographic zones. Hybrid and multi-cloud configurations can help ensure the presence of appropriate security and data geofencing needed to meet regulations and minimize security risks. To satisfy regional regulatory requirements, through 2026, nearly all multinational organizations will invest in local data processing infrastructure and services to mitigate against the risks associated with data transfer.
Our Data and Analytics Benchmark Research found that more than one-half (52%) of organizations utilize a hybrid configuration that includes on-premises and cloud infrastructure. But it is important to differentiate between large organizations “stuck with hybrid” due to piecemeal infrastructure modernization efforts, regulations, acquisitions or shadow IT, and a coordinated strategy that takes into account requirements across IT, analytics and data science. While disparate data sources and data gravity are inescapable forces, organizations harnessing data gravity with a flexible hybrid enterprise IT strategy are positioning themselves for a future competitive advantage with data science and ML.
The variable nature of data science workloads makes predicting cloud spend for AI-optimized infrastructure like GPUs a challenge, and repatriating some of these workloads from the cloud to on premises can significantly reduce TCO. If an organization is using cloud hardware and software 24/7/365, it may want to consider reallocating those workloads. Hybrid MLOps architectures allow an organization to more easily apply compute to data without having to move the data to different locations, which may lower costs, improve operational efficiency and prevent vendor lock-in.
4. Simplifying AI/ML Governance with Hybrid MLOps
Next-generation organizations should apply a hybrid strategy to MLOps, breaking down silos between environments to run data science workloads where they make the most sense based on cost, performance and regulatory considerations. These organizations implement intentional strategies to capitalize on the strengths of different environments regardless of location, while governing data science in a single system-of-record to ensure tracking, reproducibility, operational efficiency and best-practices across the enterprise.
Our Benchmark Research into Data Governance shows that 8 in 10 organizations feel governing AI/ML is important. Governing AI/ML in a connected hybrid MLOps environment does not just reduce risk through increased security, it allows data science teams to collaborate better and be more productive while still ensuring compliance with an increasing array of regulatory requirements. General Data Protection Regulation gives individuals power over the use of their personal data and holds organizations accountable for data collection and usage practices. The California Consumer Privacy Act provides similar protections specifically to California residents. The European Cloud Code of Conduct also aligns with the core elements of GDPR. And in the U.S. healthcare sector, HIPAA ensures that individuals’ health information is properly protected while allowing the flow of information needed to provide high-quality care and to protect the public’s health and well-being.
Our research also shows, 73% of organizations report that disparate data sources and systems present the greatest challenges when implementing data governance policies. Data science introduces even more challenges, requiring data from an array of sources beyond the company firewall to incorporate into analytic processes and enterprise data models. Banks, for example, access data from the branch, online and via mobile devices. Acquisitions and mergers also expand footprints in different cloud services and on-premises data centers. Collecting disparate and heterogeneous data that resides in multiple locations can complicate efforts. Beyond governance, for many IoT scenarios—particularly in storage-intensive use cases like histopathological image analysis in healthcare and life sciences exploratory research, and image, sound and vibration analysis in predictive maintenance—it does not make financial sense to move the data.
Additionally, the variable nature of data science workloads makes predicting cloud spend for AI-optimized infrastructure a challenge, and repatriating some of these workloads like computer vision and natural language processing from the cloud back to on-premises can significantly reduce TCO. Hybrid MLOps architectures allow an organization to more easily take advantage of the benefits of on-premises infrastructure which may lower costs and improve operational efficiency. At the same time, due to the unpredictable compute requirements of data science workloads, hybrid MLOps strategies should also allow for rapid scale up and out to GPUs in the cloud as needed.
5. Future-Proofing AI Strategy with a Scalable Data Science Platform
Considering the value of data and its myriad uses, organizations must carefully weigh requirements for a technology platform that efficiently converts data to valuable insights. A well-designed data science platform should prevent vendor lock-in, future-proofing your data science practice in the face of evolving hybrid strategies, ever-changing data science innovations, and maximize value from purpose-built AI infrastructure on-premises or in the cloud. The ideal platform should also accommodate the computing requirements of AI/ML, enhance data governance efforts, access distributed data, support the benefits of a hybrid architecture and cost-effectively deliver the necessary computing horsepower for advanced analytics.
Identifying computing capabilities required for evolving data science needs ensures the ability to conduct sophisticated analytics on vast amounts of data—both now and in the future. Organizations that adopt an MLOps platform supporting their hybrid enterprise IT strategy to balance openness, agility and flexible compute power to regardless of data location will gain strategic advantage.
Accelerate Enterprise Data Science in the Hybrid Cloud with MLOps
Overcome the Challenges of Operationalizing AI/ML
Data is an extremely valuable asset for every organization, but it is meaningless until it is used to make actionable decisions. Given the volume of data generated and collected today, using artificial intelligence with machine learning (AI/ML) is the most efficient way for organizations to sift through data and extract value. All industries and line-of-business functions find value by integrating AI/ML into data science efforts. Among participants in our Analytics and Data Benchmark Research, 97% of financial services organizations reported that AI/ML is important or very important, particularly for detecting and preventing fraud. Three-quarters (76%) of technology organizations also reported AI/ML is important or very important. AI/ML is often deployed to prevent cybersecurity disruptions. And more than one-half (57%) of healthcare and life sciences organizations rated AI/ML important or very important Healthcare and life science organizations use AI/ML models for numerous use cases including improving drug discoveries and creating personalized treatments to provide the best possible clinical outcomes for individuals.
However, creating, deploying and managing AI/ML models can be challenging for data and analytics executives. Our research shows that less than one in 10 organizations consider their organization’s AI/ML technology completely adequate and more than two-thirds consider it less than adequate. AI/ML presents several challenges. AI/ML requires a significant amount of data and therefore a scalable, high-performance infrastructure. It also requires a blend of data science skills with software development and operations knowledge. The tools and skills needed to code AI/ML models often do not help when dealing with the infrastructure for deploying and managing those models. Given these challenges, data and analytics executives should carefully weigh the following five considerations for AI initiatives.
1. Scaling Data Science with MLOps and GPUs
Machine learning operations (MLOps) platforms orchestrate the collection of artifacts, compute infrastructure, regardless of location, and processes necessary to deploy and maintain AI-based models. MLOps platforms facilitate close interaction between data science, application development and operations to increase productivity and deploy a greater number of models into production quickly. These platforms provide governance over access to models and the features associated with them. They help ensure models are kept up to date, detecting drift and retraining and redeploying as necessary. They also help detect and prevent bias in the models that are being developed and deployed.
Platforms that share infrastructure among multiple teams and projects help organizations enhance return on investment.
MLOps platforms also remove DevOps barriers for data scientists by providing governed access to the specialized, high-performance infrastructures powered by graphics processing units provide the horsepower needed for AI/ML workloads. GPUs, originally developed for accelerating graphics processing, provide highly parallel processing that accelerates model development and scales deployment, increasing performance and reducing cost. Given the volume of data transfers in model training, high-bandwidth memory and fast networking also help speed training iterations and time to insight.
Productivity of data science resources is critical. Platforms that share infrastructure among multiple teams and projects help organizations enhance return on investment. Increasingly, this type of specialized infrastructure is available as “chips in the cloud” as well as on-premises. While MLOps platforms are the foundation for data science at scale and can get more models into production quickly, siloed data and infrastructure often present unique challenges that disrupt the ML life cycle. This not only reduces productivity, but increases cost, wasted effort, and risk through sub-optimal model performance.
2. Managing Distributed Data On-Premises and in the Cloud
It is common for an organization to work with massive amounts of disparate, distributed data. Data is generated from business processes and gathered from systems and applications as well as sensors and devices used by the organization. Additional data is collected directly from interactions with customers, such as call logs, emails and social media posts. These more complex data sets, especially from new sources, include unstructured data, semi-structured data and external data, and must be managed across cloud services and on-premises data centers.
Through our Analytics and Data Benchmark Research, we found that more than one-half of organizations (56%) access 11 or more data sources, up from 39% four years ago. One-third of participants (32%) report using more than 20 data sources. And, as petabyte size databases become more common, more than one-half of organizations (58%) consider themselves to be using big data, up from 41% in our previous study. Understandably, nearly one-third of organizations (31%) report that it is hard to access data sources and it is the most common challenge cited in applying AI/ML.
Managed cloud services offer organizations many benefits, but on-premises data isn’t going away any time soon. Our research found that 86% of organizations either already have or expect to have the majority of data in the cloud, however on-premises deployments continue to be important to many organizations. Our research shows that more than one-half of organizations (52%) deploy their analytics and data technologies using a hybrid of on-premises and cloud platforms. By 2025, more than three-quarters of enterprises will have data spread across multiple cloud providers and on-premises data centers, requiring investment in data management products that span multiple locations.
There are several factors to consider when managing costs associated with data. Cloud providers charge ingress and egress fees, so it is no longer free to access your data. Currently, extracting and analyzing a petabyte of data could cost as much as $50,000. The cloud also requires internet connectivity to operate, which can lead to latency. Real-time analytics, databases, security applications, software connected to sensors and the IoT are subject to data gravity, creating bigger compute workloads to transmit. The split between on-premises and cloud storage can also cause price complexities.
3. Harnessing Data Gravity with a Hybrid Cloud Strategy
Data gravity is the tendency for big data to attract additional data, services and applications. The larger the data, the greater its gravity. This also relates to the data’s relative permanence, or “heaviness,” and the type of resources required to move it. While cloud architectures provide many benefits, as data grows, data gravity becomes more of an obstacle, providing new challenges for IT leaders.
Privacy regulations have renewed focus on data residency and data sovereignty, particularly with fractured data spread across multiple locations. Data residency refers to the physical location of the data, while sovereignty is the fact that data is regulated by the laws of the country in which it is collected and processed. Managing data sovereignty has become more difficult as analytic systems and data sources have become more distributed.
Through 2026, nearly all multinational organizations will invest in local data processing infrastructure and services to mitigate against the risks associated with data transfer.
Often these data sets are subject to privacy regulations meaning they must stay locked in a single geography. Cloud providers have taken steps to meet government and industry requirements, including for Health Insurance Portability and Accountability Act-compliant information, but not all providers support requirements across all geographic zones. Hybrid and multi-cloud configurations can help ensure the presence of appropriate security and data geofencing needed to meet regulations and minimize security risks. To satisfy regional regulatory requirements, through 2026, nearly all multinational organizations will invest in local data processing infrastructure and services to mitigate against the risks associated with data transfer.
Our Data and Analytics Benchmark Research found that more than one-half (52%) of organizations utilize a hybrid configuration that includes on-premises and cloud infrastructure. But it is important to differentiate between large organizations “stuck with hybrid” due to piecemeal infrastructure modernization efforts, regulations, acquisitions or shadow IT, and a coordinated strategy that takes into account requirements across IT, analytics and data science. While disparate data sources and data gravity are inescapable forces, organizations harnessing data gravity with a flexible hybrid enterprise IT strategy are positioning themselves for a future competitive advantage with data science and ML.
The variable nature of data science workloads makes predicting cloud spend for AI-optimized infrastructure like GPUs a challenge, and repatriating some of these workloads from the cloud to on premises can significantly reduce TCO. If an organization is using cloud hardware and software 24/7/365, it may want to consider reallocating those workloads. Hybrid MLOps architectures allow an organization to more easily apply compute to data without having to move the data to different locations, which may lower costs, improve operational efficiency and prevent vendor lock-in.
4. Simplifying AI/ML Governance with Hybrid MLOps
Next-generation organizations should apply a hybrid strategy to MLOps, breaking down silos between environments to run data science workloads where they make the most sense based on cost, performance and regulatory considerations. These organizations implement intentional strategies to capitalize on the strengths of different environments regardless of location, while governing data science in a single system-of-record to ensure tracking, reproducibility, operational efficiency and best-practices across the enterprise.
Our Benchmark Research into Data Governance shows that 8 in 10 organizations feel governing AI/ML is important. Governing AI/ML in a connected hybrid MLOps environment does not just reduce risk through increased security, it allows data science teams to collaborate better and be more productive while still ensuring compliance with an increasing array of regulatory requirements. General Data Protection Regulation gives individuals power over the use of their personal data and holds organizations accountable for data collection and usage practices. The California Consumer Privacy Act provides similar protections specifically to California residents. The European Cloud Code of Conduct also aligns with the core elements of GDPR. And in the U.S. healthcare sector, HIPAA ensures that individuals’ health information is properly protected while allowing the flow of information needed to provide high-quality care and to protect the public’s health and well-being.
Our research also shows, 73% of organizations report that disparate data sources and systems present the greatest challenges when implementing data governance policies. Data science introduces even more challenges, requiring data from an array of sources beyond the company firewall to incorporate into analytic processes and enterprise data models. Banks, for example, access data from the branch, online and via mobile devices. Acquisitions and mergers also expand footprints in different cloud services and on-premises data centers. Collecting disparate and heterogeneous data that resides in multiple locations can complicate efforts. Beyond governance, for many IoT scenarios—particularly in storage-intensive use cases like histopathological image analysis in healthcare and life sciences exploratory research, and image, sound and vibration analysis in predictive maintenance—it does not make financial sense to move the data.
Additionally, the variable nature of data science workloads makes predicting cloud spend for AI-optimized infrastructure a challenge, and repatriating some of these workloads like computer vision and natural language processing from the cloud back to on-premises can significantly reduce TCO. Hybrid MLOps architectures allow an organization to more easily take advantage of the benefits of on-premises infrastructure which may lower costs and improve operational efficiency. At the same time, due to the unpredictable compute requirements of data science workloads, hybrid MLOps strategies should also allow for rapid scale up and out to GPUs in the cloud as needed.
5. Future-Proofing AI Strategy with a Scalable Data Science Platform
Considering the value of data and its myriad uses, organizations must carefully weigh requirements for a technology platform that efficiently converts data to valuable insights. A well-designed data science platform should prevent vendor lock-in, future-proofing your data science practice in the face of evolving hybrid strategies, ever-changing data science innovations, and maximize value from purpose-built AI infrastructure on-premises or in the cloud. The ideal platform should also accommodate the computing requirements of AI/ML, enhance data governance efforts, access distributed data, support the benefits of a hybrid architecture and cost-effectively deliver the necessary computing horsepower for advanced analytics.
Identifying computing capabilities required for evolving data science needs ensures the ability to conduct sophisticated analytics on vast amounts of data—both now and in the future. Organizations that adopt an MLOps platform supporting their hybrid enterprise IT strategy to balance openness, agility and flexible compute power to regardless of data location will gain strategic advantage.
Fill out the form to continue reading
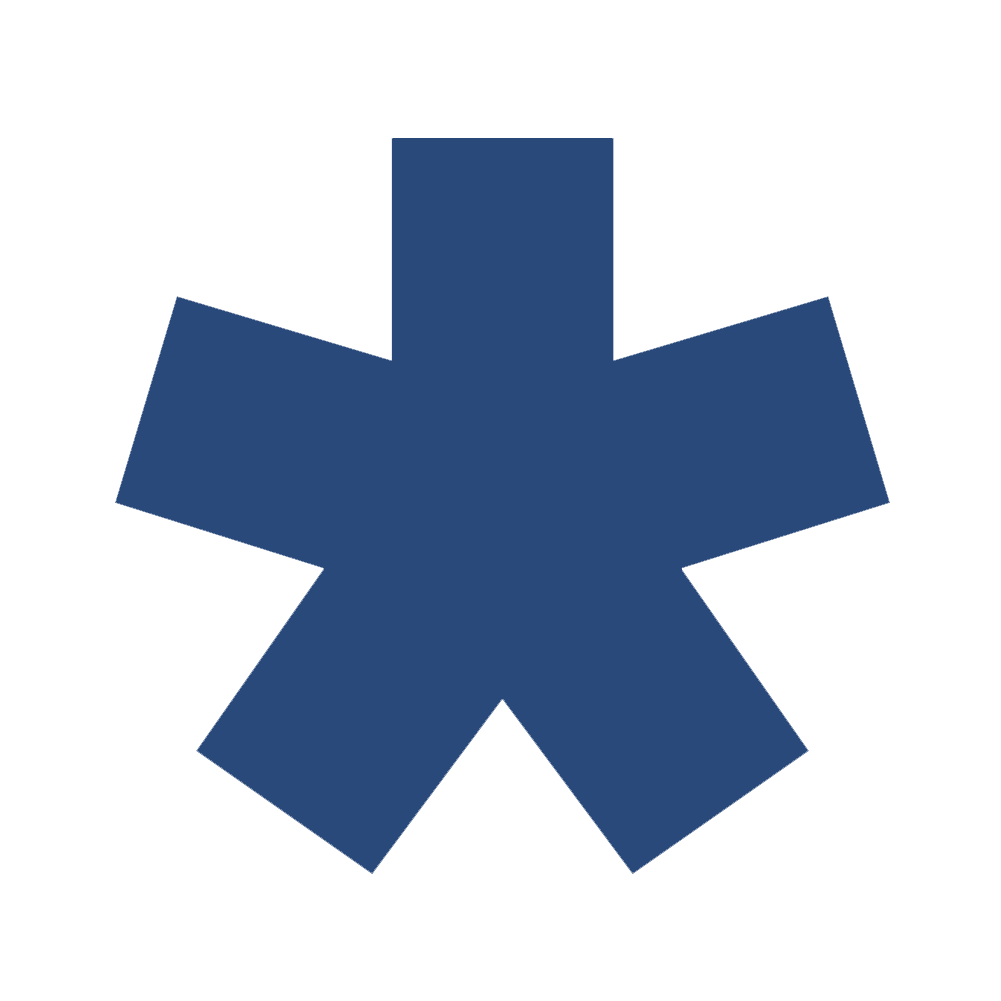
ISG Software Research
ISG Software Research is the most authoritative and respected market research and advisory services firm focused on improving business outcomes through optimal use of people, processes, information and technology. Since our beginning, our goal has been to provide insight and expert guidance on mainstream and disruptive technologies. In short, we want to help you become smarter and find the most relevant technology to accelerate your organization's goals.
About ISG Software Research
ISG Software Research provides expert market insights on vertical industries, business, AI and IT through comprehensive consulting, advisory and research services with world-class industry analysts and client experience. Our ISG Buyers Guides offer comprehensive ratings and insights into technology providers and products. Explore our research at www.isg-research.net.
About ISG Research
ISG Research provides subscription research, advisory consulting and executive event services focused on market trends and disruptive technologies driving change in business computing. ISG Research delivers guidance that helps businesses accelerate growth and create more value. For more information about ISG Research subscriptions, please email contact@isg-one.com.
About ISG
ISG (Information Services Group) (Nasdaq: III) is a leading global technology research and advisory firm. A trusted business partner to more than 900 clients, including more than 75 of the world’s top 100 enterprises, ISG is committed to helping corporations, public sector organizations, and service and technology providers achieve operational excellence and faster growth. The firm specializes in digital transformation services, including AI and automation, cloud and data analytics; sourcing advisory; managed governance and risk services; network carrier services; strategy and operations design; change management; market intelligence and technology research and analysis. Founded in 2006 and based in Stamford, Conn., ISG employs 1,600 digital-ready professionals operating in more than 20 countries—a global team known for its innovative thinking, market influence, deep industry and technology expertise, and world-class research and analytical capabilities based on the industry’s most comprehensive marketplace data.
For more information, visit isg-one.com.