Read Time:
7 min.
Sponsored by:
Font Size:
Font Weight:
Closing the Loop on Data Generation and Business Decisions
Accelerating Business Insight
The current economic climate has highlighted more than ever the differences between organizations that can turn data into actionable insights and those that are incapable of seeing or responding to the need for change. Data-driven organizations stand to gain a competitive advantage when they increase the speed and scale of how data is accessed and processed and ensure both the security and accuracy of that data.
Organizations can fall into the trap of trying to create the perfect data repository to deliver a single version of the truth across the business.
It remains the case that, even in a data-driven organization, a high proportion of data and analytics projects fail to deliver on expectations. There are multiple reasons why these initiatives fail. A delicate balance of people, process, information and technology is required to deliver a successful IT project, and small deviations in any one of those factors can send projects off the rails. For data projects specifically, organizations can fall into the trap of trying to create the perfect data repository to deliver a single version of the truth across the business. The over-emphasis on traditional approaches to data processing and analytics results in organizations spending far too much time preparing and transforming data to make it suitable for the selected repository, delaying actionable insight.
Vendors are addressing the speed and accuracy with which data is acquired from enterprise applications and delivered to end users, but these applications and data warehouse or lake environments are substantial investments that can be hard to modernize due to cost, complexity and scale. Organizations stand to benefit from focusing less on architecting and constructing data repositories and pipelines and instead paying closer attention to achieving desired outcomes that deliver measurable business value from the data.
Emphasizing Outcomes Over Data Architecture
For data-driven organizations to gain—and maintain—a competitive advantage, data must be processed quickly, accurately and at a large volume. End users and data consumers need to be able to confidently use data to make better decisions faster, which frees up time to innovate with more advanced initiatives, including predictive analytics and artificial intelligence (AI). Up-to-the-minute refreshes lead to more accurate predictions and forecasts. Efficiency, accuracy and security are key elements to success.
Giving line-of-business departments ready access to accurate and up-to-the-minute data organized in the way they need it increases productivity. Efficiency leads to optimized costs, improved revenue and customer experience, and supports an organization’s innovation, growth and scale. Global media corporations, for example, that focus on streaming and aggregation need data processed quickly, accurately and securely so that the various departments within the enterprise can effectively service millions of customers. Workers also need to be able to slice and dice the information into whatever form best suits their needs at that moment rather than sifting through the details or transforming the data.
A platform that allows organizations to securely aggregate a large volume of data and analyze it in its raw, untransformed structure enables faster, more accurate decisions. Legacy applications are unlikely to be tossed out, but organizations do not need to abandon existing applications and systems to benefit. Adding a complementary platform to existing architectures enables companies to get more value from their current technology while giving them the ability to, over time, condense their technology spend.
Data Preparation Delays Business Insight
Data preparation is essential to ensuring that business decisions are based on high-quality data. Unfortunately, it is also hugely time-consuming. Our Analytics and Data Benchmark Research found that 69% of organizations spend most of their time in analytic initiatives preparing data. Legacy ERP applications require data to be extracted, transformed and loaded into analytic data platforms before it can be analyzed, but the process of reformatting and transforming data introduces unnecessary costs and errors and reduces agility. Data in enterprise applications is highly normalized to reduce redundancy and improve integrity. Transforming and restructuring data as it is loaded into a data warehouse based on known analytic patterns reduces the ability to rapidly respond to changing data and business requirements. There is also a risk that data becomes stuck in operational or analytic silos, resulting in the failure of organizations to realize value from their investments.
Time spent reviewing the prepared data for errors and inconsistencies creates additional delays in providing actionable information to executives and managers. In fact, data preparation efforts consume so much time that only 27% of organizations can spend the bulk of their time focusing on how changes are affecting the business. These delays can prevent organizations from achieving the agility and resiliency necessary in today’s environment, resulting in missed opportunities, increased costs and heightened risks. Technical debt built up by the failure to rectify data preparation and data quality delays can lead to additional opportunity costs as fixing the problem requires an investment of time and resources that could be better spent on initiatives that deliver actionable insight.
Legacy applications limit scale and speed. Traditional applications are focused on running the business based on operational data, which is extracted and combined in data warehouses or data lakes to create reports and dashboards used to analyze the business. This results in business decision-makers having at least two sources of information that must be sorted through. Employees need access to data pertinent to their role to facilitate a data-centric culture, preferably via analytics embedded in operational applications that present data to users in a format that aligns with their business workflow and objectives.
Expedited Delivery of Business-Ready Data
To be successful, staff must have immediate, easy access to the right data in the right format. Preprocessing data in its natural form retains the application data models and facilitates responsiveness to changing data and business requirements. Retaining data in its natural form enables the use of pre-packaged data applications that combine best practices, schemas and analytic dashboards focused on specific functional areas, including financial consolidation and planning, spend control and supply chain planning. Many organizations, especially larger ones, have ERP systems from multiple vendors or multiple instances with different chart of accounts configurations. Ventana Research uses the term “data pantry” to describe a system that ensures all the necessary data ingredients are within easy reach of business domains, such as the office of finance, and with labels that are easily understood.
The data pantry all but eliminates time spent on data preparation and the need to check for accuracy. Managing data continuously from end-to-end in a process ensures its quality. A hands-off, technology-based approach to moving data provides greater control and is necessary because any break in this chain introduces the possibility of errors. It also means that data is available sooner and in whatever detail is necessary.
Our Analytics and Data Benchmark Research found that only 22% of organizations analyze their data in real time. Reducing the lag between data collection and data analysis accelerates business decision making. Data connectors, parallel data loading, schema loading and schema inspection capabilities to pre-process data as it is loaded help determine potential query paths and accelerate query performance. Increasing the number of data rows improves timely access, while maintaining the state of data helps to avoid bottlenecks. The ability to process large volumes of raw data avoids the limitations of having to deal with aggregated views. Incremental refreshes of ERP data directly to a dashboard or report allows for a faster ROI versus traditional or legacy applications in which insight is delayed while data is bulk loaded and then transformed to meet the requirements of the data warehouse, and only presented to decision makers when—or if—the related reports and dashboards are refreshed.
Supporting Intelligent Applications with Predictive and Generative AI
In addition to dashboards and reports, an increasing proportion of organizations are taking advantage of AI to support intelligent applications. Nearly nine in 10 organizations use or plan to adopt some form of AI technology to remain competitive in their respective markets. Organizations need a combination of generative AI and predictive AI to gain a full and accurate picture of their business opportunities and challenges.
Predictive analytics uses statistical and machine learning (ML) algorithms to analyze historical data and make accurate and detailed predictions about the future. It does so by identifying patterns, relationships and trends in the data to forecast future outcomes. Predictive analytics requires a continuous cycle of data collection and preparation, model building and testing, and deployment and ongoing testing of the model. Our Office of Finance Benchmark Research found that only 24% of organizations utilize predictive analytics to any degree, likely because they rely on manual data collection and preparation which, for many using legacy applications, is too time consuming to be practical. Automating this step and continuously updating the data pantry with a broad array of operational, financial and external data make actionable detailed and accurate predictive analytics possible.
The emergence of generative AI and large language models (LLM) have accelerated enthusiasm about the new ways in which AI can be applied and has the potential to transform many aspects of how individuals interact with technology of all types. LLMs may be the breakthrough needed to make natural language processing (NLP) much more widely used. We expect the adoption of generative AI to grow rapidly, asserting that through 2025, one-quarter of organizations will deploy generative AI embedded in one or more software applications.
The ability to trust the output of generative AI models will be critical to adoption by enterprises. Content generated by current LLMs is occasionally incoherent and incorrect. It can include factual inaccuracies, such as fictitious data and source references. There are multiple approaches that organizations can take to reduce trust and accuracy concerns. These fall into two categories. The first is Retrieval Augmented Generation (RAG), where pre- and in-process approaches include training and fine-tuning open-source models with enterprise data, augmenting open-source and proprietary models with context from enterprise content and data, and prompt engineering to shape and refine responses. Second, organizations can employ post-process approaches that include automated and human validation of data to ensure that the output of LLMs are consistent with enterprise data.
Next Steps
There are multiple approaches available on the market for accelerating analysis of data, especially in cloud-based data lakes. Typically, these are positioned as platforms that require the replacement of the previous generation of technology in order to expedite business processes and the generation of actionable insight. When looking to accelerate business decision-making, organizations should look for opportunities that emphasize speed, accessibility, accuracy and the business value of embracing change. Vendors offering these platforms this should emphasize the value of complementing existing applications and platforms, provide business users with access to data and insight in the applications and workflows used to run the business, and identify how their application reduces and closes the loop on the generation of data and the formulation of business decisions based on that data.
Closing the Loop on Data Generation and Business Decisions
Accelerating Business Insight
The current economic climate has highlighted more than ever the differences between organizations that can turn data into actionable insights and those that are incapable of seeing or responding to the need for change. Data-driven organizations stand to gain a competitive advantage when they increase the speed and scale of how data is accessed and processed and ensure both the security and accuracy of that data.
Organizations can fall into the trap of trying to create the perfect data repository to deliver a single version of the truth across the business.
It remains the case that, even in a data-driven organization, a high proportion of data and analytics projects fail to deliver on expectations. There are multiple reasons why these initiatives fail. A delicate balance of people, process, information and technology is required to deliver a successful IT project, and small deviations in any one of those factors can send projects off the rails. For data projects specifically, organizations can fall into the trap of trying to create the perfect data repository to deliver a single version of the truth across the business. The over-emphasis on traditional approaches to data processing and analytics results in organizations spending far too much time preparing and transforming data to make it suitable for the selected repository, delaying actionable insight.
Vendors are addressing the speed and accuracy with which data is acquired from enterprise applications and delivered to end users, but these applications and data warehouse or lake environments are substantial investments that can be hard to modernize due to cost, complexity and scale. Organizations stand to benefit from focusing less on architecting and constructing data repositories and pipelines and instead paying closer attention to achieving desired outcomes that deliver measurable business value from the data.
Emphasizing Outcomes Over Data Architecture
For data-driven organizations to gain—and maintain—a competitive advantage, data must be processed quickly, accurately and at a large volume. End users and data consumers need to be able to confidently use data to make better decisions faster, which frees up time to innovate with more advanced initiatives, including predictive analytics and artificial intelligence (AI). Up-to-the-minute refreshes lead to more accurate predictions and forecasts. Efficiency, accuracy and security are key elements to success.
Giving line-of-business departments ready access to accurate and up-to-the-minute data organized in the way they need it increases productivity. Efficiency leads to optimized costs, improved revenue and customer experience, and supports an organization’s innovation, growth and scale. Global media corporations, for example, that focus on streaming and aggregation need data processed quickly, accurately and securely so that the various departments within the enterprise can effectively service millions of customers. Workers also need to be able to slice and dice the information into whatever form best suits their needs at that moment rather than sifting through the details or transforming the data.
A platform that allows organizations to securely aggregate a large volume of data and analyze it in its raw, untransformed structure enables faster, more accurate decisions. Legacy applications are unlikely to be tossed out, but organizations do not need to abandon existing applications and systems to benefit. Adding a complementary platform to existing architectures enables companies to get more value from their current technology while giving them the ability to, over time, condense their technology spend.
Data Preparation Delays Business Insight
Data preparation is essential to ensuring that business decisions are based on high-quality data. Unfortunately, it is also hugely time-consuming. Our Analytics and Data Benchmark Research found that 69% of organizations spend most of their time in analytic initiatives preparing data. Legacy ERP applications require data to be extracted, transformed and loaded into analytic data platforms before it can be analyzed, but the process of reformatting and transforming data introduces unnecessary costs and errors and reduces agility. Data in enterprise applications is highly normalized to reduce redundancy and improve integrity. Transforming and restructuring data as it is loaded into a data warehouse based on known analytic patterns reduces the ability to rapidly respond to changing data and business requirements. There is also a risk that data becomes stuck in operational or analytic silos, resulting in the failure of organizations to realize value from their investments.
Time spent reviewing the prepared data for errors and inconsistencies creates additional delays in providing actionable information to executives and managers. In fact, data preparation efforts consume so much time that only 27% of organizations can spend the bulk of their time focusing on how changes are affecting the business. These delays can prevent organizations from achieving the agility and resiliency necessary in today’s environment, resulting in missed opportunities, increased costs and heightened risks. Technical debt built up by the failure to rectify data preparation and data quality delays can lead to additional opportunity costs as fixing the problem requires an investment of time and resources that could be better spent on initiatives that deliver actionable insight.
Legacy applications limit scale and speed. Traditional applications are focused on running the business based on operational data, which is extracted and combined in data warehouses or data lakes to create reports and dashboards used to analyze the business. This results in business decision-makers having at least two sources of information that must be sorted through. Employees need access to data pertinent to their role to facilitate a data-centric culture, preferably via analytics embedded in operational applications that present data to users in a format that aligns with their business workflow and objectives.
Expedited Delivery of Business-Ready Data
To be successful, staff must have immediate, easy access to the right data in the right format. Preprocessing data in its natural form retains the application data models and facilitates responsiveness to changing data and business requirements. Retaining data in its natural form enables the use of pre-packaged data applications that combine best practices, schemas and analytic dashboards focused on specific functional areas, including financial consolidation and planning, spend control and supply chain planning. Many organizations, especially larger ones, have ERP systems from multiple vendors or multiple instances with different chart of accounts configurations. Ventana Research uses the term “data pantry” to describe a system that ensures all the necessary data ingredients are within easy reach of business domains, such as the office of finance, and with labels that are easily understood.
The data pantry all but eliminates time spent on data preparation and the need to check for accuracy. Managing data continuously from end-to-end in a process ensures its quality. A hands-off, technology-based approach to moving data provides greater control and is necessary because any break in this chain introduces the possibility of errors. It also means that data is available sooner and in whatever detail is necessary.
Our Analytics and Data Benchmark Research found that only 22% of organizations analyze their data in real time. Reducing the lag between data collection and data analysis accelerates business decision making. Data connectors, parallel data loading, schema loading and schema inspection capabilities to pre-process data as it is loaded help determine potential query paths and accelerate query performance. Increasing the number of data rows improves timely access, while maintaining the state of data helps to avoid bottlenecks. The ability to process large volumes of raw data avoids the limitations of having to deal with aggregated views. Incremental refreshes of ERP data directly to a dashboard or report allows for a faster ROI versus traditional or legacy applications in which insight is delayed while data is bulk loaded and then transformed to meet the requirements of the data warehouse, and only presented to decision makers when—or if—the related reports and dashboards are refreshed.
Supporting Intelligent Applications with Predictive and Generative AI
In addition to dashboards and reports, an increasing proportion of organizations are taking advantage of AI to support intelligent applications. Nearly nine in 10 organizations use or plan to adopt some form of AI technology to remain competitive in their respective markets. Organizations need a combination of generative AI and predictive AI to gain a full and accurate picture of their business opportunities and challenges.
Predictive analytics uses statistical and machine learning (ML) algorithms to analyze historical data and make accurate and detailed predictions about the future. It does so by identifying patterns, relationships and trends in the data to forecast future outcomes. Predictive analytics requires a continuous cycle of data collection and preparation, model building and testing, and deployment and ongoing testing of the model. Our Office of Finance Benchmark Research found that only 24% of organizations utilize predictive analytics to any degree, likely because they rely on manual data collection and preparation which, for many using legacy applications, is too time consuming to be practical. Automating this step and continuously updating the data pantry with a broad array of operational, financial and external data make actionable detailed and accurate predictive analytics possible.
The emergence of generative AI and large language models (LLM) have accelerated enthusiasm about the new ways in which AI can be applied and has the potential to transform many aspects of how individuals interact with technology of all types. LLMs may be the breakthrough needed to make natural language processing (NLP) much more widely used. We expect the adoption of generative AI to grow rapidly, asserting that through 2025, one-quarter of organizations will deploy generative AI embedded in one or more software applications.
The ability to trust the output of generative AI models will be critical to adoption by enterprises. Content generated by current LLMs is occasionally incoherent and incorrect. It can include factual inaccuracies, such as fictitious data and source references. There are multiple approaches that organizations can take to reduce trust and accuracy concerns. These fall into two categories. The first is Retrieval Augmented Generation (RAG), where pre- and in-process approaches include training and fine-tuning open-source models with enterprise data, augmenting open-source and proprietary models with context from enterprise content and data, and prompt engineering to shape and refine responses. Second, organizations can employ post-process approaches that include automated and human validation of data to ensure that the output of LLMs are consistent with enterprise data.
Next Steps
There are multiple approaches available on the market for accelerating analysis of data, especially in cloud-based data lakes. Typically, these are positioned as platforms that require the replacement of the previous generation of technology in order to expedite business processes and the generation of actionable insight. When looking to accelerate business decision-making, organizations should look for opportunities that emphasize speed, accessibility, accuracy and the business value of embracing change. Vendors offering these platforms this should emphasize the value of complementing existing applications and platforms, provide business users with access to data and insight in the applications and workflows used to run the business, and identify how their application reduces and closes the loop on the generation of data and the formulation of business decisions based on that data.
Fill out the form to continue reading
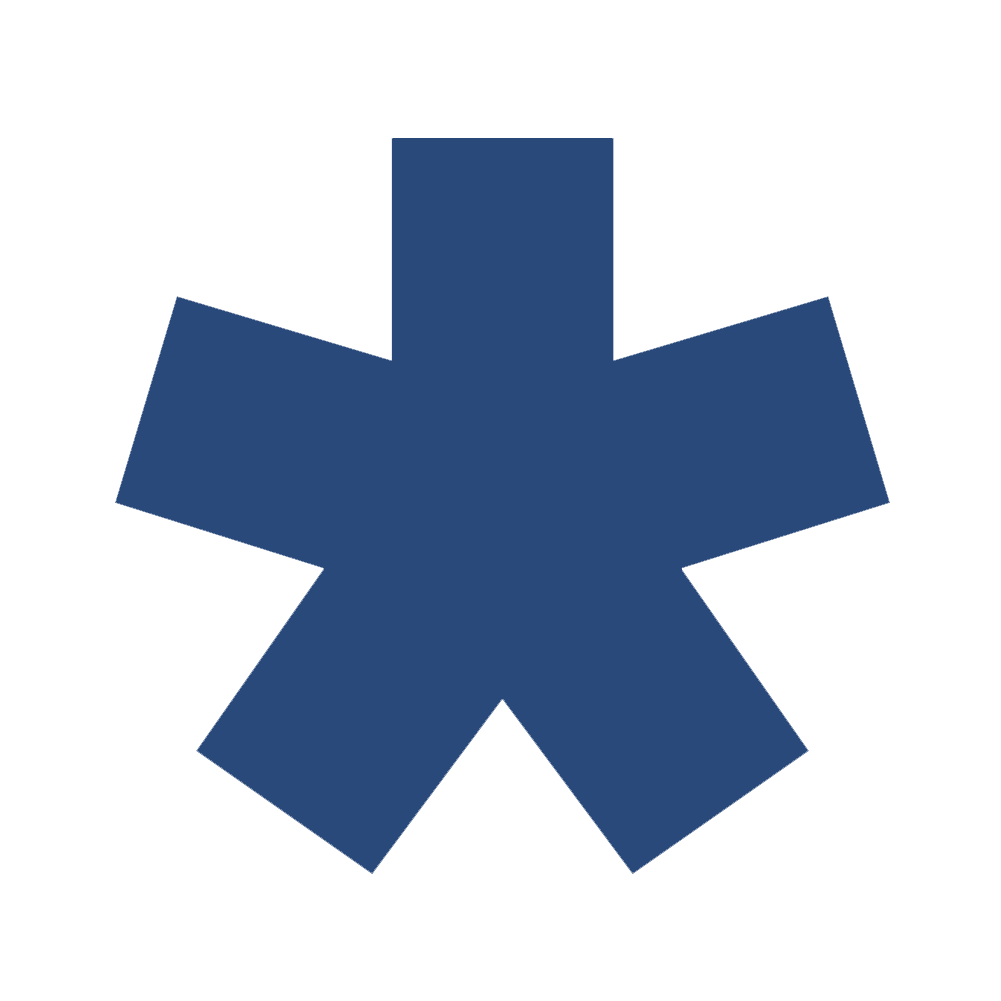
ISG Software Research
ISG Software Research is the most authoritative and respected market research and advisory services firm focused on improving business outcomes through optimal use of people, processes, information and technology. Since our beginning, our goal has been to provide insight and expert guidance on mainstream and disruptive technologies. In short, we want to help you become smarter and find the most relevant technology to accelerate your organization's goals.
About ISG Software Research
ISG Software Research provides expert market insights on vertical industries, business, AI and IT through comprehensive consulting, advisory and research services with world-class industry analysts and client experience. Our ISG Buyers Guides offer comprehensive ratings and insights into technology providers and products. Explore our research at www.isg-research.net.
About ISG Research
ISG Research provides subscription research, advisory consulting and executive event services focused on market trends and disruptive technologies driving change in business computing. ISG Research delivers guidance that helps businesses accelerate growth and create more value. For more information about ISG Research subscriptions, please email contact@isg-one.com.
About ISG
ISG (Information Services Group) (Nasdaq: III) is a leading global technology research and advisory firm. A trusted business partner to more than 900 clients, including more than 75 of the world’s top 100 enterprises, ISG is committed to helping corporations, public sector organizations, and service and technology providers achieve operational excellence and faster growth. The firm specializes in digital transformation services, including AI and automation, cloud and data analytics; sourcing advisory; managed governance and risk services; network carrier services; strategy and operations design; change management; market intelligence and technology research and analysis. Founded in 2006 and based in Stamford, Conn., ISG employs 1,600 digital-ready professionals operating in more than 20 countries—a global team known for its innovative thinking, market influence, deep industry and technology expertise, and world-class research and analytical capabilities based on the industry’s most comprehensive marketplace data.
For more information, visit isg-one.com.